Víctor Guallar: "AI will have to be sustainable in the future or we won’t have a future"
Barcelona Supercomputing Center (BSC)
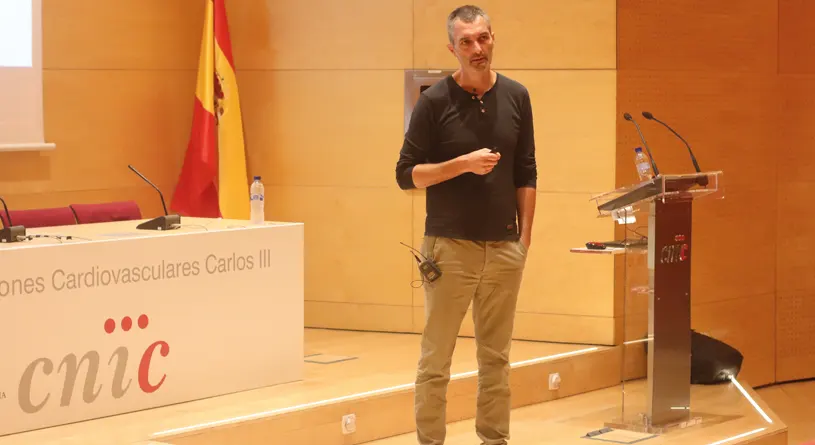
Victor Guallar is ICREA Professor at the Barcelona Supercomputing Centre (BSC). He obtained his doctorate in theoretical chemistry between the Autonomous University of Barcelona (Spain) and University of California, Berkeley (USA). After three years as a postdoctoral researcher at the University of Columbia in New York, he was appointed assistant professor at the University of Washington’s Medical School before transferring his group to the BSC in 2006. His laboratory (EAPM) makes major contributions to computational biophysics and biochemistry. He has been awarded various research projects, including an advanced ERC grant (the youngest researcher to have received one in Spain). He is also founder of the first BSC spin-off business, Nostrum Biodiscovery, a young biotechnology company created in 2016 with the goal of collaborating with pharmaceutical and biotech companies devoted to developing medicines and molecules of biotech interest.
Artificial intelligence (AI) heralds a pharmacological revolution, what does it mean?
We’re in the face of a revolution. Now, from three basic ingredients, we can find molecules for any target and the find very good molecules or potential drugs that can inhibit it. Before, you were very lucky if you found one or two molecules and it took six months or a year to do so. Now, in a month we identify 100 molecules, of which 20 or 30 are very good. What has changed research so much? I think three fundamental things have changed.
First, we now have very large virtual libraries. Until recently, we had a library of 5 or 10 million, and now we have virtual libraries that we use on a daily basis of 6 billion. We have just signed an agreement with a company to access a library of 34 billion and we hope to have another of 48 billion. Most of these libraries are free access. They are virtual libraries because many of the molecules don’t exist, but in all of the projects we’ve worked on with them, 80% of the molecules are available in less than a month at a very reasonable price, 100 or 150 dollars per molecule. And, with artificial intelligence, we have infinite space because it’s the only tool that can search in such large libraries. Traditional classic techniques cannot search in such a large space because they aren’t designed to do so, we’d take months. AI enables us to search in such a large space in a matter of hours, for instance, in 12 hours we can search the 6 billion.
And AI also offers the possibility of generating new molecules. So, from molecules that appear to be good, we can ask AI to search for 5000 completely new molecules that do not exist, that nobody has invented before, because they are related as they are small variations. But although AI enables the search for molecules in libraries of 6 billion, it makes a lot of mistakes. It is here that traditional molecular modelling techniques, which have improved year on year, help us refine the search and guide AI to search in a more approximate direction. Then, we apply Active Learning, where AI makes a prediction, classic molecular modelling researches the prediction and tells it, you were wrong, improve it. It is a cycle of improvement. We do 5 or 6 cycles in a week, and we have a list of molecules that in all projects have up to 70% success. It’s spectacular.
All pharmaceutical companies are looking for new targets
What’s missing? Targets for these molecules: give me a target and we’ll find the compounds. nother thing that AI has given us are the structures of the targets. This allows us to search for molecules in a little under a month, whereas before it took us 2 years. And a great variety. Before, if you were lucky, you found one or two molecules Now, as we are searching within 6 billion, we find 20 or 30 completely different molecules that are very good inhibitors of the target. So, what is the real problem facing pharmacology? Before, you would find 2 or 3 molecules, but 90% failed during the clinical phase. Now, we have 20, although not all of them will reach the clinical phase. With the combination of knowledge about drug development and AI, the search will become refined. Another aspect that is faster is the experimental research phase in the laboratory. With AI we gain about 18 months and, above all, diversity of candidates. It really is a very substantial improvement Right now; the bottleneck is targets. Phenotypes and pharmacological targets. That means, if I touch this, I cure that. All pharmaceutical companies are looking for new targets.
So do you think the way in which medicines are manufactured will change?
No, they’ll be made in the same way, but in terms of cost, they’ll be cheaper because there will be a higher success rate at later stages. Investments of so many millions of dollars won’t happen, or if they do, it will be with a higher success rate. The clinical phases will cost what they cost, but the success rate will not be 20%, it will be 30% or 40%. We’ve already been working on this for two years. For instance, with Clotet Bonaventura’s group at IrsiCaixa, we have a vaccine for COVID developed using supercomputing. We are now using the same technique to work for Hipra and we are developing vaccines for new targets.
For any disease?
Yes, in principle, although what we have set up is for viruses like COVID. But a great deal of progress is being made in immunology at computational level in selecting which part of the antigen is going to enable production of a much better vaccine, in finding neoantigens, and now we are working on selecting the patient’s T cells that could be the ones to give an immunological response. Lots of work is being done with in silico testing.
Although there are still people who don’t believe in computation, in 10 years there won’t be anything in a laboratory that has not been AI tested
Centres like yours will be indispensable.
Right now, we’re overwhelmed. Although we’ve been working in this field for many years, the current success rate has made it a very valuable tool. Now we can be confident we’ll find a molecule, or we’ll improve a vaccine a lot, thanks to supercomputing. Although there are still people who don’t believe in computation, in 10 years there won’t be anything in a laboratory that has not been AI tested. Everything that reaches the laboratory will have been preselected, which will mean gains in time and efficiency.
How do you see the future of supercomputing?
I’m very concerned about climate change. Society should know that AI contributes to contamination of the planet, and in two or three years it may become one of the main producers of emissions. It has to be made sustainable: the people who use AI to play should be aware that they are producing contamination. They have to be told that there is a server working in a place that uses electricity, which produces emissions. And there are many researchers working on sustainable AI. AI will have to be sustainable in the future or we won’t have a future. And demand is going to increase heavily. Now, in my laboratory everything we do involves AI.
How can this situation be controlled?
As a society, we have to take unpopular measures that may restrict, a little, our freedom; that could be the only way to ensure our children’s future on Earth. I’m not very optimistic, although with the COVID pandemic, for instance, we have a unique example showing that as a society we can stop for a few months and find a solution to a problem. The thing is, people don’t see climate change, overpopulation or contamination as killers. But they are.
Society should know that AI contributes to contamination of the planet, and in two or three years it may become one of the main producers of emissions
How long have you been interested in computation?
When I was a little boy, I wanted to be a biochemist, but I wasn’t good in the laboratory, and I liked machines. I remember that when the first computers came out, at home we didn’t have the money to buy one, and I read books about computers. I always liked programming, etc.
Did you think it would develop as it has?
Yes. I was clear that it would be predictive.
And of all the developments, is AI the most important?
No, it isn’t a whole: the ordered growth of simulation techniques, computational capacity. Without large servers, AI could do nothing.